Transforming Business Operations with a Machine Learning Labeling Tool
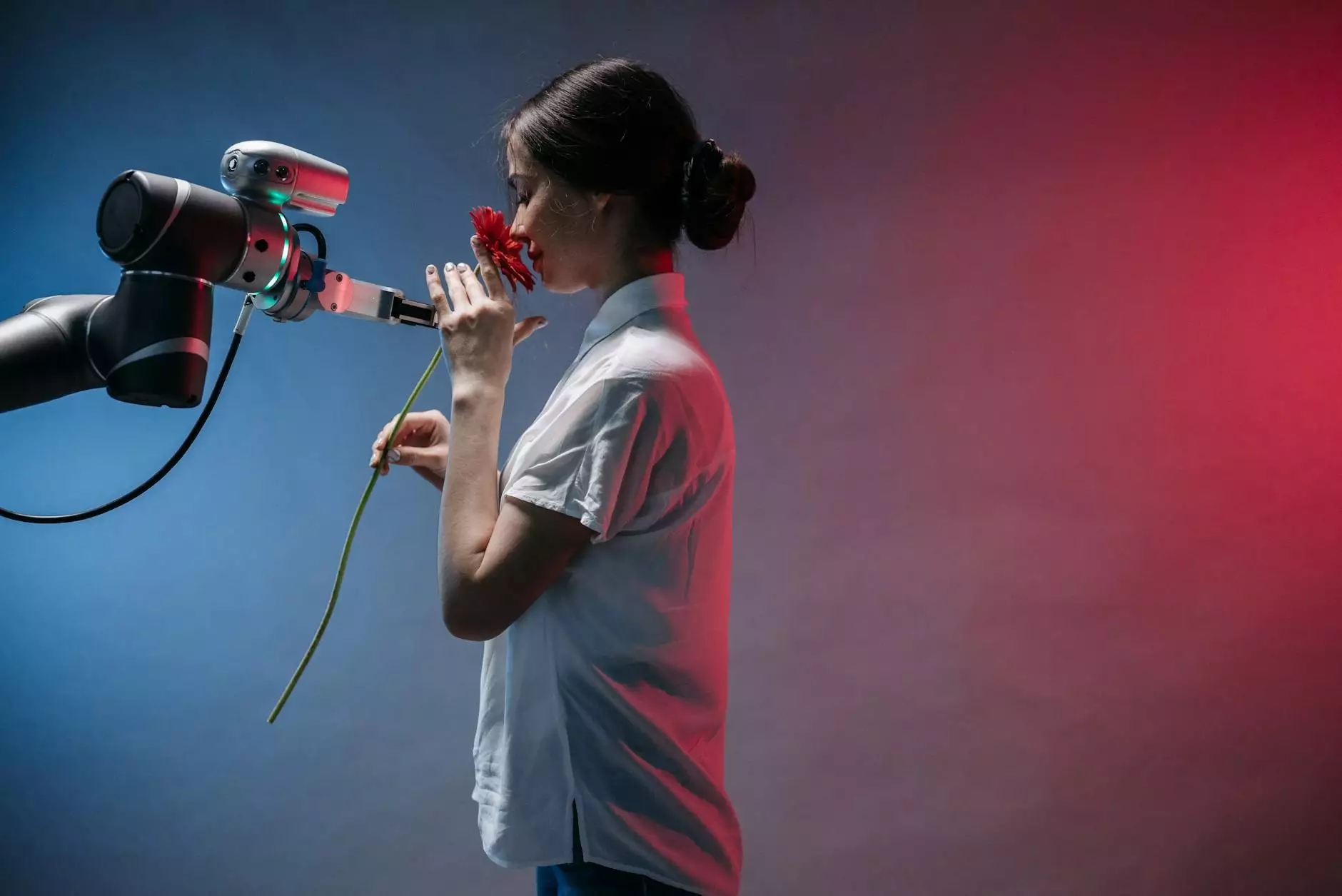
In today's digital era, businesses are inundated with data. The ability to harness this data effectively can mean the difference between success and stagnation. One of the critical aspects of managing data effectively is through labeling, particularly in the context of machine learning. A machine learning labeling tool is an innovative solution that systematically optimizes this process and enables organizations to unlock the true potential of their data. This article will delve deeply into how such tools can be a game changer for businesses, especially within the realm of software development.
The Importance of Data Labeling in Machine Learning
Data is an invaluable asset, driving insights that can enhance decision-making and operational efficiency. However, raw data on its own is often unstructured and lacks contextual meaning. This is where data labeling becomes essential.
Data labeling involves the process of annotating data so that machine learning models can learn from it. For instance, in image recognition tasks, images need to be labeled with relevant tags like “dog”, “cat”, or “car” so that the model can make accurate predictions. The quality and accuracy of these labels can significantly impact the performance of machine learning algorithms.
What is a Machine Learning Labeling Tool?
A machine learning labeling tool is a specialized software solution designed to assist organizations in the data labeling process. It provides a framework conducive to annotating various forms of data, including images, audio files, and text documents, ensuring that the data used for training machine learning models is both high-quality and comprehensive. These tools often leverage advanced features such as:
- Automated Labeling: Some tools utilize pre-trained models to provide automated labeling suggestions, reducing the time and effort required for human annotators.
- User-Friendly Interfaces: Many machine learning labeling tools are designed with intuitive interfaces that make it easy for users to manage and annotate data.
- Collaboration Features: These tools often allow multiple users to work on labeling tasks simultaneously, streamlining workflow and improving productivity.
- Quality Assurance: Advanced tools incorporate quality assurance mechanisms to verify the accuracy of labels, ensuring only reliable training data is used.
Key Benefits of Implementing a Machine Learning Labeling Tool
Investing in a machine learning labeling tool yields numerous benefits, including:
1. Enhanced Accuracy and Efficiency
Manually labeling data is often a tedious process prone to human error. Machine learning labeling tools automate significant portions of this process, resulting in higher accuracy overall. This efficiency not only saves time but also reduces operational costs.
2. Scalability
As businesses grow, so do their datasets. A robust labeling tool scales seamlessly to accommodate increasing data, ensuring that organizations can keep pace with their data needs without sacrificing quality.
3. Streamlined Workflows
Collaboration features in machine learning labeling tools facilitate teamwork, allowing multiple stakeholders to contribute to the labeling process. This reduces bottlenecks and ensures faster project turnaround times.
4. Improved Model Performance
The primary goal of any machine learning model is to achieve high performance. By ensuring that the data fed into these models is accurately labeled, organizations can improve their model's predictive capabilities, leading to better business outcomes.
Choosing the Right Machine Learning Labeling Tool
With numerous labeling tools available, selecting the right one for your business is critical. Here are essential criteria to consider:
- Types of Data Supported: Ensure the tool supports the types of data you will be working with, whether it be images, text, or audio.
- Integration Capabilities: The tool should easily integrate with your existing workflow and software ecosystem.
- Customization Options: Look for tools that allow you to customize labels and workflows to fit your specific needs.
- Cost Efficiency: Consider both upfront costs and long-term value, including the impact on team productivity.
The Role of Keymakr in Your Business
Keymakr, as a key player in the realm of software development, offers state-of-the-art solutions, including effective machine learning labeling tools tailored to enhance business operations. By leveraging Keymakr's expertise, organizations can enhance their data labeling processes, ensuring reliability, quality, and scalability.
With solutions designed for both small startups and large enterprises, Keymakr helps businesses seamlessly navigate the complexities of data labeling while focusing on core objectives like innovation and growth. Keymakr's commitment to quality and customer satisfaction is evident in its comprehensive support and user-friendly interfaces.
Real-World Applications of Machine Learning Labeling Tools
The applications of machine learning labeling tools are vast and varied, spanning multiple industries. Here are some notable examples:
1. Healthcare
In the healthcare sector, machine learning labeling tools are utilized to annotate medical images, aiding in the early detection and diagnosis of diseases. Tools help radiologists by providing accurate labels for conditions, leading to improved patient outcomes.
2. Autonomous Vehicles
Self-driving technology relies heavily on labeled data from video feeds and sensor input. Machine learning labeling tools enable automotive companies to streamline the annotation of data necessary for refining their algorithms.
3. E-commerce
In the e-commerce arena, labeling tools assist in product categorization and image tagging, enhancing the customer shopping experience and boosting sales through improved searchability.
4. Natural Language Processing
In text-based applications, such as chatbots and sentiment analysis, machine learning labeling tools facilitate entity recognition and intent classification, which are crucial for providing accurate responses to user queries.
Future Trends in Machine Learning Labeling Tools
The landscape of machine learning labeling tools is continuously evolving. Here are some trends to watch for in the coming years:
- Integration with AI: Future labeling tools will likely incorporate more AI capabilities to automate and optimize the labeling process further.
- Increased Use of Collaborative Labeling: Enhanced collaborative features will foster team-based approaches to data labeling.
- Focus on Data Privacy and Security: As data privacy becomes paramount, tools will increasingly incorporate features that ensure the secure handling of sensitive information.
- Expansion of Pre-trained Models: The use of pre-trained models will gain traction, providing users with quick labeling solutions for less complex datasets.
Conclusion
In conclusion, the advancements in machine learning labeling tools are transforming the way businesses approach data. By adopting a robust machine learning labeling tool, organizations can enhance their operational efficiency, improve the performance of their machine learning models, and ultimately drive growth.
Whether your business is in healthcare, automotive, e-commerce, or any other sector, the right labeling tool can streamline data processes and yield significant benefits. Partnering with reliable providers like Keymakr ensures that you are equipped with the best tools in the industry, paving the way for innovative solutions and successful outcomes.
Invest in a machine learning labeling tool today and take the first step towards revolutionizing your data management strategy!